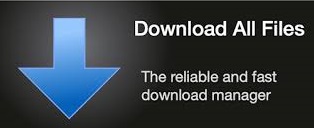

The opposite team gains a certain amount of points by exterminating the other team, this is an effective way to gain points but it is hard to achieve if there's many players. In this mode you'll also trigger a team perk if your team gets a certain amount of kills, your team will be blessed with some helpful skill for a certain amount of seconds.Ī PvP gamemode, solely for humans.CyberCon, the notorious global corporation, aspired to deliver to the world something that would change the fate of humanity. Their schemes had been controversial to most before, but their newest idea seemed almost too perfect.Ī much sinister secret was about to be exposed. CyberCon's project involved the development of neuro-hub chips. They succeeded and became a phenomenon, later becoming a mandatory law to have them implanted at birth using state of the art surgery and cutting edge technology.ĬyberCon were granted this permission from the government. The controversy was covered up and very little was known how it was done. The technology was seen by many around the world as a major breakthrough that could cure blindness, deafness, disease, and a huge boost in performance for modern day life. It was further aiding as a gateway to easier communication and enhanced capabilities. This was the next milestone in human history. Your browser does not support the video = ,Īll the broadcast videos are provided courtesy of the Tennis Channel.įor any question regarding this research paper, please contact Haotian Zhang via adapted from GLAMR.A global profile system/leveling system.Extreme amounts of gore & gibbing, this is your lovely grindhouse-gore movie!.Over 20 unique weapons, including akimbo weapons!.However, good things must always come to an end.Īnd CyberCon's true colors are yet to be revealed. Right-hand, one-handed backhand (far) View 1 Left-hand, two-handed backhand (near) vs. Right-hand, two-handed backhand (far) View 1 Right-hand, one-handed backhand (near) vs. Your browser does not support the video tag. Overall, our system can synthesize two physically simulated characters playing extended tennis rallies with simulated racket and ball dynamics. We demonstrate that our system produces controllers for physically-simulated tennis players that can hit the incoming ball to target positions accurately using a diverse array of strokes (serves, forehands, and backhands), spins (topspins and slices), and playing styles (one/two-handed backhands, left/right-handed play). To address the low quality of motions extracted from broadcast videos, we correct estimated motion with physics-based imitation, and use a hybrid control policy that overrides erroneous aspects of the learned motion embedding with corrections predicted by the high-level policy. When deployed at scale on large video collections that encompass a vast set of examples of real-world tennis play, our approach can learn complex tennis shotmaking skills and realistically chain together multiple shots into extended rallies, using only simple rewards and without explicit annotations of stroke types. Our approach is built upon hierarchical models, combining a low-level imitation policy and a high-level motion planning policy to steer the character in a motion embedding learned from broadcast videos. We present a system that learns diverse, physically simulated tennis skills from large-scale demonstrations of tennis play harvested from broadcast videos.
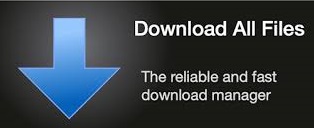